Winnonlin Trial Download
WinNonlin ® Version 5.2 WinNonlin ® Version 5.2 WinNonlin copyright ©1998-2007 Pharsight Corporation.
- To download PHOENIX WINNONLIN. But with the benefit of the free trial, at least you phoenix winnonlin see for yourself whether or not it's worth the investment.
- Pharmacokinetic Software. David@boomer.org Whenever you download a file from the Internet please. A free, fully-functional, trial copy and a detailed.
Associated Data
Abstract
In order to help companies qualify and validate the software used to evaluate bioequivalence trials with two parallel treatment groups, this work aims to define datasets with known results. This paper puts a total 11 datasets into the public domain along with proposed consensus obtained via evaluations from six different software packages (R, SAS, WinNonlin, OpenOffice Calc, Kinetica, EquivTest). Insofar as possible, datasets were evaluated with and without the assumption of equal variances for the construction of a 90% confidence interval. Not all software packages provide functionality for the assumption of unequal variances (EquivTest, Kinetica), and not all packages can handle datasets with more than 1000 subjects per group (WinNonlin). Where results could be obtained across all packages, one showed questionable results when datasets contained unequal group sizes (Kinetica). A proposal is made for the results that should be used as validation targets.
Electronic supplementary material
The online version of this article (doi:10.1208/s12248-014-9704-6) contains supplementary material, which is available to authorized users.
INTRODUCTION
Bioequivalence testing is a general requirement for companies developing generic medicines, testing food effects, making formulation changes, or developing extensions to existing approved medicines where absorption rate and extent to the systemic circulation determines safety and efficacy. Throughout many countries and jurisdictions, the common way of testing for bioequivalence is to compare the pharmacokinetics of the new formulation (“test”) with that of the known formulation (“reference”). Using non-compartmental analysis, the primary metrics derived in bioequivalence studies are most often the area under the concentration time curve until the last sampling point (AUCt) and the maximum observed concentration (Cmax) for both the test and the reference. A confidence interval is then constructed on basis of two one-sided t tests, typically at a nominal α level of 5%. The most common designs for bioequivalence testing are the two-treatment, two-sequence, two-period randomized crossover design and the randomized two-group parallel design. The former is considerably more common than the latter and the design of choice for active ingredients whose half-lives are not prohibitively long (1–4).
To evaluate the data obtained in a bioequivalence trial, companies must use validated software but in the absence of datasets with known results, it is difficult to actually know if the software acquired correctly performs the task it is supposed to do and therefore, it is practically impossible to validate software in-house beyond installation qualification and operational qualification. On that basis, we recently published a paper in this journal with reference datasets for the two-treatment, two-sequence, two-period crossover trials and where the datasets were evaluated with different software packages (5).
Since trials with two parallel groups are the second most common type of bioequivalence studies and published datasets with known results are scarce, the purpose of this paper is to propose reference datasets for two-group parallel trials and derive 90% confidence intervals with different statistical software packages in order to establish consensus results that can be used—together with the datasets—to qualify or validate software analyzing the outcomes from parallel group bioequivalence trials. It is outside the scope of this paper to discuss more than two groups or the other design options that exist such as replicate designs.
For validation purposes, datasets should be of varying complexity in terms of imbalance, outliers, range, heteroscedasticity, and point estimate in order to cover any situation which can reasonably be expected to occur in practice. It is not the aim of this work to validate any software or to advocate for or against any specific software package.
MATERIALS AND METHODS
Datasets
The datasets used in this paper seek to include small and large data sets, outliers, unequal group sizes, and heteroscedasticity as a type of stress test. The characteristics of datasets are as follows:
This is the data of the first period from Clayton and Leslie (6) with balance between treatment groups, i.e., equal sizes of the test treatment (NT) and the reference treatment (NR): NT = NR = 9.
Based on dataset P1, where subjects 10…14 were removed (NT = 9, NR = 4).
Based on dataset P1, where the raw data entry for subject 4 has been multiplied by 100.
This is a dataset simulated on basis of a log-normal distribution, where the simulated geometric means ratio (GMR) was 0.95 with balance between treatment groups (NT = NR = 20) and heteroscedasticity (CVT = 0.25 and CVR = 2.5).
This is a dataset simulated on basis of a log-normal distribution, where the simulated GMR was 1.1 and with slight imbalance (NT = 31, NR = 29) and homoscedasticity (CVR = CVT = 0.05). Although a CV of 0.05 is not realistic for any drug tested in humans; variabilities at this level are often seen with orally inhaled products (e.g., delivered dose). Applicants in Europe may choose the parallel bioequivalence model for their testing. To qualify for approval on basis of such data, the actual requirement is a 90% confidence interval with a 15% equivalence margin corresponding to an acceptance range of 85.00–117.65% when a multiplicative model is used (7).
This is the dataset from Bolton and Bon (8); NT = 24, NR = 26.
This is a dataset simulated on basis of a log-normal distribution, where the simulated GMR was 1.2 with imbalance between treatment groups (NT = 1000 and NR = 200) and heteroscedasticity (CVT =0.25 and CVR = 2.5).
This is a dataset simulated on basis of a log-normal distribution, where the simulated GMR was 1.1 and with balance between treatment groups (NT = NR = 1000) and homoscedasticity (CVR = CVT = 0.5).
This is a dataset simulated on basis of a log-normal distribution, where the simulated GMR was 1.1 and with balance between treatment groups (NT = 1000, NR = 1,000) and heteroscedasticity (CVR = 0.25, CVT = 2.5).
Based on dataset P6, where the raw data entry for subject 138 (receiving test) was multiplied by 100.
Based on dataset P6, where the raw data entries for subjects 1…50 (test) and subjects 1001…1050 (reference) were multiplied by 100,000.
All datasets can be downloaded as tab-delimited text files as “Electronic Supplementary Material” from the journal’s homepage. The supplementary material also presents individual box-plots of the datasets.
Evaluation of Datasets
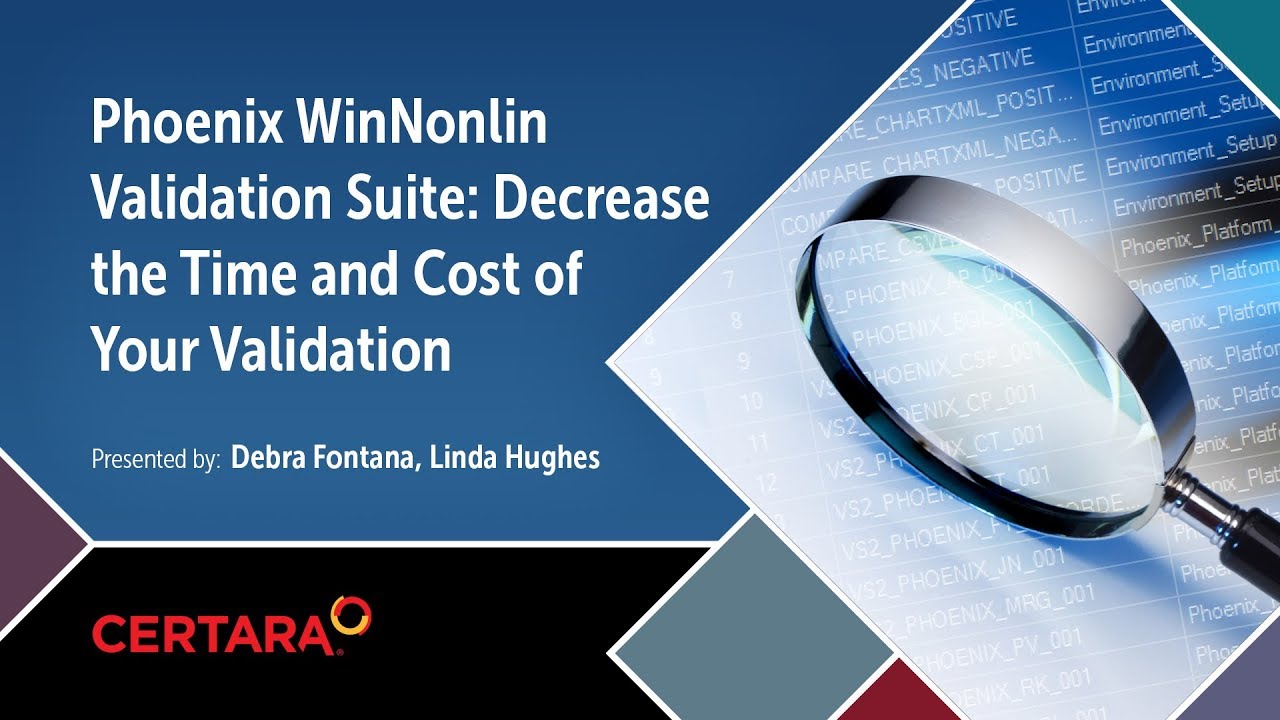
The datasets were evaluated with
R (version 3.0.2, R Foundation for Scientific Computing 2013), running under Windows 8 (64 bit).
OpenOffice Calc (version 4.1.0, The Apache Software Foundation 2014), running under Windows 8. A spreadsheet was set up.
Phoenix/WinNonlin (64-bit version 6.4.0.768, Pharsight, A Certara Company 2014), running under Windows 7 (64 bit).
EquivTest/PK (Statistical Solutions 2006), running in a 32-bit XP Virtual PC under Windows 7 (64 bit).
Kinetica (version 5.0.10, Thermo Fisher Scientific 2007), running in a 32-bit XP Virtual PC under Windows 7 (64 bit).
SAS (32 bit version 9.2, SAS Institute 2008), running under Windows 7 (64 bit).
The datasets were evaluated with and without the Welch correction for degrees of freedom (ν), corresponding to either assuming heteroscedasticity (unequal variances) or homoscedasticity (equal variances), respectively, of treatment groups.
In the Welch correction, the degrees of freedom are approximated as
where sT2 and sR2 are the variances, and NT and NR are the sample sizes of groups treated by the test and reference, respectively.
If no Welch correction is applied the degrees of freedom are
In all cases, datasets were evaluated towards construction of a 90% confidence interval (CI) around the ratio of log-transformed sample means (point estimate, PE), corresponding to a nominal α level of 5%
where t1 − α,ν is the one-sided critical value of Student’s t distribution at ν degrees of freedom for the given α (in this paper 0.05) and MSE is the mean square error (estimated pooled variance).
R and SAS are script-/code-based applications. Examples of scripts used for these two software packages are uploaded as a supplementary material. They should be adapted to the user’s preferences. The OpenOffice Calc spreadsheet is also uploaded as a supplementary material.
Phoenix/WinNonlin natively does not offer evaluations based on the Welch correction. A workaround (9,10) was used in generating results given in Table TableI.I. The Welch correction appears to be impossible in the menu-driven software packages EquivTest/PK and Kinetica.
Table I
Results obtained with the different statistical packages with Welch correction
Dataset | 90% confidence interval (point estimate) | |||
---|---|---|---|---|
R | OO Calc | WinNonlin | SAS | |
P1 | 26.78–88.14 (48.58) | 26.78–88.14 (48.58) | 26.78–88.14 (48.58)a | 26.78–88.14 (48.58) |
P2 | 23.71–74.38 (41.99) | 23.71–74.38 (41.99) | 23.71–74.38 (41.99)a | 23.71–74.38 (41.99) |
P3 | 24.40–449.08 (104.67) | 24.40–449.08 (104.67) | 24.40–449.08 (104.67)a | 24.40–449.08 (104.67) |
P4 | 38.05–136.15 (71.97) | 38.05–136.15 (71.97) | 38.05–136.15 (71.97)a | 38.05–136.15 (71.97) |
P5 | 106.44–112.10 (109.23) | 106.44–112.10 (109.23) | 106.44–112.10 (109.23)a | 106.44–112.10 (109.23) |
P6 | 91.84–115.79 (103.12) | 91.84–115.79 (103.12) | 91.84–115.79 (103.12)a | 91.84–115.79 (103.12) |
P7 | 97.38–138.51 (116.14) | 97.38–138.51 (116.14) | b | 97.38–138.51 (116.14) |
P8 | 105.79–113.49 (109.57) | 105.79–113.49 (109.57) | b | 105.79–113.49 (109.57) |
P9 | 103.80–120.61 (11.89) | 103.80–120.61 (11.89) | b | 103.80–120.61 (11.89) |
P10 | 97.82–139.17 (116.68) | 97.82–139.17 (116.68) | b | 97.82–139.17 (116.68) |
P11 | 6.30–21.60 (11.67) | 6.30–21.60 (11.67) | b | 6.30–21.60 (11.67) |
Ninety percent confidence intervals and point estimates are given in percent of the reference rounded to two decimals as suggested by the FDA and EMA. Calculation apparently not possible in EquivTest/PK and Kinetica
aResults obtained by the workaround (9)
bNot applicable due to the software’s limitations
RESULTS
Table TableII presents the 90% confidence intervals with point estimates evaluated by the different software packages if equal variances are not assumed (i.e., the Welch correction is applied).
Table TableIIII presents the corresponding results if equal variances are assumed.
Table II
Results obtained with the different statistical packages without Welch correction
Dataset | 90% confidence interval (point estimate) | |||||
---|---|---|---|---|---|---|
R | OO Calc | WinNonlin | EquivTest | Kinetica | SAS | |
P1 | 27.15–86.94 (48.58) | 27.15–86.94 (48.58) | 27.15–86.94 (48.58) | 27.15–86.94 (48.58) | 27.15–86.94 (48.58) | 27.15–86.94 (48.58) |
P2 | 18.26–96.59 (41.99) | 18.26–96.59 (41.99) | 18.26–96.59 (41.99) | 18.26–96.59 (41.99) | 15.76–119.00 (41.99) | 18.26–96.59 (41.99) |
P3 | 26.35–415.71 (104.67) | 26.35–415.71 (104.67) | 26.35–415.71 (104.67) | 26.35–415.71 (104.67) | 26.35–415.71 (104.67) | 26.35–415.71 (104.67) |
P4 | 38.60–134.21 (71.97) | 38.60–134.21 (71.97) | 38.60–134.21 (71.97) | 38.60–134.21 (71.97) | 38.60–134.21 (71.97) | 38.60–134.21 (71.97) |
P5 | 106.44–112.10 (109.23) | 106.44–112.10 (109.23) | 106.44–112.10 (109.23) | 106.44–112.10 (109.23) | 106.39–112.14 (109.23) | 106.44–112.10 (109.23) |
P6 | 91.85–115.78 (103.12)a | 91.85–115.78 (103.12)a | 91.85–115.78 (103.12)a | 91.85–115.78 (103.12)a | 92.07–115.50 (103.12) | 91.85–115.78 (103.12)a |
P7 | 106.86–126.23 (116.14) | 106.86–126.23 (116.14) | 106.86–126.23 (116.14) | 106.86–126.23 (116.14) | 104.30–129.32 (116.14) | 106.86–126.23 (116.14) |
P8 | 105.79–113.49 (109.57) | 105.79–113.49 (109.57) | 105.79–113.49 (109.57) | 105.79–113.49 (109.57) | 105.79–113.49 (109.57) | 105.79–113.49 (109.57) |
P9 | 103.80–120.61 (111.89) | 103.80–120.61 (111.89) | 103.80–120.61 (111.89) | 103.80–120.61 (111.89) | 103.80–120.61 (111.89) | 103.80–120.61 (111.89) |
P10 | 107.20–126.99 (116.68) | 107.20–126.99 (116.68) | 107.20–126.99 (116.68) | 107.20–126.99 (116.68) | 104.59–130.16 (116.68) | 107.20–126.99 (116.68) |
P11 | 7.83–17.38 (11.67) | 7.83–17.38 (11.67) | 7.83–17.38 (11.67) | 7.83–17.38 (11.67) | 6.98–19.51 (11.67) | 7.83–17.38 (11.67) |
Ninety percent confidence intervals and point estimates are given in percent of the reference rounded to two decimals as suggested by the FDA and EMA
aAccords with Bolton and Bon (8)
DISCUSSION
The conventional t test is fairly robust against violations of homoscedasticity but quite sensitive to unequal group sizes (11). Furthermore, preliminary testing for equality of variances is flawed and should be avoided (12). If assumptions are violated, the t test becomes liberal, i.e., the patient’s risk might exceed the nominal level and an alternative (e.g., based on the Welch correction) is suggested (11,13). FDA’s guidance states: “For parallel designs […] equal variances should not be assumed” (14).
In WinNonlin, a somewhat cumbersome workaround allows a Welch correction for construction of the confidence interval, whereas in EquivTest/PK and Kinetica, there appears to be no option to evaluate two-group parallel designs with the Welch correction for unequal variances. Due to limitations of WinNonlin (fixed factors are restricted to 1000 levels), we were not able to process datasets P7–P11 with the published workaround.
Kinetica consistently arrived at confidence intervals that differed from the other packages if datasets had unequal group sizes (i.e., P2, P5–7, P10–P11). A similar phenomenon was noted in a previous publication presenting balanced and imbalanced reference datasets for two-treatment, two-sequence, two-period crossover bioequivalence trials (5). Although we do not have access to Kinetica’s source code, it is noted that when group sizes are equal (that is, when NT = NR), Eq. (3) can be simplified to
and if this version of the equation uncritically is applied to imbalanced datasets, then
The point estimate remains correct.
The width of the confidence interval will change. The use of Eq. (4) could therefore increase the chance of products appearing approvable (confidence intervals narrower) when NT < NR and could decrease the chance of products appearing approvable (confidence intervals wider) when NT > NR .
The results coincide with Kinetica’s that we found for the investigated datasets. Equation (4) is stated in the user manual (15).
While it is outside the scope of this paper to specifically debate any specific software’s general fitness for bioequivalence evaluations, the fact that different packages arrive at different results with identical datasets underscore the need for proper validation. The datasets and evaluations presented here are relatively simple; in various areas of drug development, much more sophisticated statistical models with many more numerical settings are available. Examples include mixed effect models used for longitudinal data or replicated bioequivalence trials, and survival or time-to-event trials evaluated by Kaplan-Meier derivatives, to mention but a few. To help companies qualify or validate their software for such evaluations, we call for other authors to publish datasets of varying complexity with known results that can be confirmed by multiple statistical packages.
CONCLUSION
This paper releases 11 datasets into the public domain along with proposed consensus results in order to help companies qualify and validate their statistical software for evaluation of bioequivalence trials with two treatment groups. The datasets are evaluated with different statistical packages with and without the assumption of equal variances for the construction of the 90% confidence interval. Not all packages are able to use a Welch correction for unequal variances when analyzing the datasets and not all packages can handle the largest datasets. In addition, one package seemed to arrive at results that stand in contrast to others. We propose the results obtained with R, which are identical to those obtained with SAS (and WinNonlin, where possible) to be targeted by companies qualifying or validating their software. The datasets are available as supplementary material.
Electronic Supplementary Material
Contributor Information
Anders Fuglsang, Email: moc.amrahpgnaslguf@ufna.
Helmut Schütz, Phone: +43 12311746, Email: ta.cabeb@zteuhcs.tumleh.
Detlew Labes, Phone: +49 3342237973, Email: moc.gadrdcc@sebald.